A Concise Guide to Recruitment Chatbots in 2024

This article will discover how these AI marvels are setting new benchmarks in talent acquisition, making recruitment smarter, faster, and more attuned to the needs of the modern workforce. In today’s competitive job market, maintaining open communication with candidates is essential for fostering engagement and building employer brand reputation. Recruitment chatbots serve as virtual assistants, providing timely updates chatbot for recruiting on application statuses, scheduling interviews, and slot gacor hari ini answering frequently asked questions. By integrating chat widgets into career websites and job portals, organizations can offer instant support to candidates, enhancing their overall experience and increasing the likelihood of successful hires. Over the last 10 years, most larger companies have posted jobs on job boards, with links to apply on a corporate career site.

Capable of handling large numbers of applicants simultaneously, chatbots are particularly effective in large-scale recruitment drives. Their scalability ensures that even during high-volume periods, the recruitment process remains smooth and efficient. Throughout the recruiting process, recruiters often take on tasks that are necessary but don’t add value for candidates. Chatbots can allow recruiters to spend more time with the strongest candidates by taking on some of the administrative tasks.
There’s a reason you’ve probably come across every recruitment chatbot in this list – they’re either the best (like, ahem, Sense), or they spend an awful lot on Google ads
. Our Recruitment Chatbot feature in ATS will help you engage with talent 24/7, providing prompt replies to standard questions. Almost every industry nowadays uses chatbots for different purposes, such as hospitality, E-commerce, healthcare, education, information & technology, financial and legal, and recruitment. Further, since employees access it through the tools they already use for collaboration (Slack and Teams, for instance), engagement rates for customers have been known to spike after MeBeBot’s swift implementation. Three key factors on which we compare these HR chatbot tools are the AI engine behind the conversational interface, the user-friendliness of the interaction, and its automation capabilities. As with any purchase, it’s important to consider your budget when selecting a recruiting chatbot.
Increase your conversions with chatbot automation!
They assess candidates purely based on skills and qualifications, supporting equal-opportunity hiring. Chatbots have become much more advanced in the past few years, as natural language processing continues to improve. Much of the evolution is due to the improved technology that can read and respond more naturally to candidates. Efficiency is a game-changer in recruitment, and AI Chatbots have proved to be invaluable tools in streamlining the hiring process. Try building your very own recruitment chatbot today and bring your talent acquisition into the modern era of digital experiences. Job boards are saturated with job offers with companies looking and ready to fight for the best talent they can get.
Also, it gives an impression of the innovative and modern company culture that attracts more candidates. On the other hand, the ROI of HR chatbots is 100% about time savings with hiring and recruiting. We recently talked to HR thought leader Bennet Sung, who suggested that the internal effect of these tools is massive. Based on his years of experience, he shared that the most common use case for HR chatbots is self-service automation for FAQs from employees. The chatbot can also help interviewers schedule interviews, manage feedback, and alert candidates as they progress through the hiring process.
Will Chatbots Take Over HR Tech? Paradox Sets The Pace. – Josh Bersin
Will Chatbots Take Over HR Tech? Paradox Sets The Pace..
Posted: Thu, 04 Apr 2024 07:00:00 GMT [source]
During the hiring process, candidates invariably have many questions, ranging from job responsibilities and compensation to benefits and application procedures. Recruitment chatbots step in here, providing quick and accurate responses to these frequently asked questions. Available 24/7, they ensure that candidates can receive timely answers outside of standard business hours, enhancing the overall candidate experience. In a market where the right talent is akin to finding a needle in a haystack, recruitment chatbots are the magnets drawing skilled professionals to the right roles. They’re not just tools for efficiency; they’re bridges between opportunity and talent, ensuring that the recruitment process is no longer a daunting task for HR teams or a frustrating journey for candidates.
As AI and machine learning algorithms become more sophisticated, chatbots will become even more intelligent and capable of handling complex tasks. Future advancements may include the ability of chatbots to conduct video interviews, simulate real-life work scenarios to assess candidates’ skills, and even predict the success of a candidate in a particular role. These enhancements will further streamline the hiring process and ensure that companies make informed decisions when selecting candidates. Furthermore, chatbots may also be integrated with social media platforms and job boards, allowing companies to reach potential candidates where they spend most of their time online. This broadens the scope of talent acquisition and provides companies with access to a more diverse pool of candidates.
Improve your customer experience within minutes!
A recruiting chatbot is a sophisticated tool that leverages HR analytics and integrates with recruitment management systems (RMS) to offer advanced functionalities, automating various stages of the recruitment process. Recruitment chatbots can effectively administer employee referral programs, making it easy for staff to refer candidates and track the status of their referrals. Chatbots can be programmed to eliminate bias in the screening process, ensuring fairness and diversity in candidate selection.
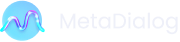
If you choose your questions smartly, you can easily weed out the applications that give HR managers headaches. So, in case the minimum required conditions are not met, you can have the bot inform the applicant that unfortunately, they are not eligible for the role right on the spot. Even if you are already working with a certain applicant tracking system, you can use Landbot to give your application process a human touch while remaining efficient. These simple steps allow you to screen through applications efficiently focusing on candidates with the right type or years of experience and qualifications. However, you can always create new ones to serve any personalized purpose as we created above, just so you can get going creating an interactive chatbot resume.
What major companies are using HR and recruiting chatbots?
Now, Upwage’s immediate plans involve scaling rapidly and effectively to meet the demands of its growing user base. Facebook Groups and Facebook-promoted posts are generating applicants for many employers. But, Once a candidate gets to your Facebook Careers Page, what are they supposed to do? With an automated Messenger Recruitment Chatbot, candidates can “Send a Message” to the Facebook page chatbot. The Messenger chatbot can then engage the candidate, ask for their profile information, show them open jobs, and videos about working at your company, and even create Job Alerts, over Messenger. Below are some recruitment chatbot examples to help you understand how recruiting chatbots can help, what they can do, and ways to implement them.
Best Recruitment & HR Chatbots to Automate With – Employee Benefit News
Best Recruitment & HR Chatbots to Automate With.
Posted: Mon, 08 Apr 2024 07:00:00 GMT [source]
Gone are the days of manually sifting through mountains of resumes to identify the right candidate. These intelligent virtual assistants have transformed the way employers screen candidates, personalize the recruitment experience, improve efficiency, reduce bias, and even impact recruiters’ roles. One such cutting-edge AI Chatbot is Iris, an AI Talent Scout that automates sourcing, shortlisting, and candidate outreach, significantly enhancing the effectiveness and speed of the recruitment process.
In addition, this artificial intelligence can also ask questions about experience and interests to prequalify those seeking employment. They can also answer questions that an applicant may have about the job search and schedule a time for an individual to speak with a recruiter. Chatbots aid in onboarding new hires by providing essential information, guiding them through initial paperwork, and answering basic queries. This support makes the onboarding experience smoother and more welcoming for new employees.
Recruitment chatbots offer transformative benefits for the talent acquisition process, enhancing efficiency, candidate experience, and operational effectiveness. However, the adoption of this technology should be approached with a clear understanding of its limitations and the need for ongoing development and oversight. By balancing these factors, businesses can leverage recruitment chatbots to their fullest potential, ensuring a more streamlined and effective recruitment process.

By automating routine recruitment tasks, chatbots free HR staff to concentrate on strategic elements of talent acquisition. This shift from administrative duties to more impactful areas of recruitment strategy amplifies the effectiveness of the HR team. Chatbots offer immediate, round-the-clock responses to applicant inquiries, significantly enhancing the candidate experience. This constant availability and interaction foster a positive perception of the company, keeping candidates engaged and informed throughout the recruitment journey.
To make sure that the technology can effectively communicate, employers should look for a chatbot that is part of a larger technology solution that works throughout the entire application process. No more one-size-fits-all approaches; these messages are carefully tailored to explain precisely why a candidate is an excellent match for the position. As a result, candidates feel valued and appreciated throughout the hiring process.
Turn applications into easy conversations, ask knock-out questions, and integrate with your ATS. More than a standard chatbot, our platform is powered by natural language processing for seamless interactions. As a standalone chatbot; however, AllyO performs as you would hope and expect a recruiting chatbot to function, allowing candidates to ask questions, schedule interviews, and prescreen for a particular position. Their integrations list; however, is underwhelming (and again lacks the most common ATSs for our friends in staffing & recruiting). Hence, By responding immediately, Chatbots engage with their users and increase candidate engagement. In addition, the recruitment bot collects basic information such as the name, email ID, resume, and answers to the pre-screening questions from the applicants.
Moreover, they expand the candidate pool by considering individuals with diverse perspectives and experiences who might not fit traditional molds but bring fresh insights to the role. Additionally, AI assesses professional achievements and skills beyond what’s typically required for a particular position, offering a more dynamic perspective of each candidate’s potential. With AI Chatbots handling these mundane activities, recruiters can focus on building relationships with top candidates, conducting more insightful interviews, and making well-informed hiring decisions. Dialpad Ai Virtual Assistant is our solution that leverages conversational AI for self-service interactions. Dialpad is also an omnichannel platform, meaning it lets your recruiters talk to candidates (and each other) through a whole range of communication channels—all in one place. But having to constantly input new data and workflows can be pretty high-effort (and potentially costly).
They simplify and accelerate the screening and selection of candidates, improve the candidate experience, attract top talent, and offer valuable insights to both companies and job seekers. As technology continues to advance, we can expect even more exciting advancements in recruitment chatbot technology, further enhancing the benefits they bring to the recruitment and hiring processes. It’s like having an extra team member who works around the clock, tirelessly sorting through applications, scheduling interviews, and even assisting in initial candidate screening. These chatbots use advanced algorithms, machine learning, and natural language processing to interact in a way that feels surprisingly human.
AI technology helps in this filtering process of matching jobs as per the uploaded resume by the candidates. As a result, many staffing agencies and large recruitment firms started using this AI-powered talent acquisition tool to improve the candidate experience in the recruitment process. HR chatbots are automated conversational agents that assist in recruiting and HR tasks, engaging with candidates, answering inquiries, and streamlining processes. They can take care of repeatable and straightforward functions so that your HR staff are freed up to concentrate on higher-level assignments. A seamless and engaging recruitment process, facilitated by chatbots, positively reflects on the employer’s brand. It demonstrates a commitment to innovation and candidate experience, attracting top talent.
In addition, candidates have come to expect a consumer-like application and hiring experience that is similar to other interactions they’re having online and on their smartphones every day. One way that self-service tools can be used in talent acquisition and recruitment is by automating the initial screening process. This means that rather than having a recruiter or HR Manager manually review each application (which can be incredibly time-consuming), a recruitment bot can be used to do this instead.
These tasks can be voice requests, like asking Siri or Google Assistant to look up information, or can be a candidate responding to a job ad over text messaging. After using the hiring bot in the recruitment workflow, VBZ started to experience following positive changes. Recruitment Chatbot utilisation and adaptation have increased in the recruitment landscape as the trend of virtual recruiting started booming after the COVID-19 pandemic. To run your own numbers, feel free to download our ROI calculator for HR and Recruiting chatbots. For a tailored quote aligned with your company’s dimensions, you’ll need to arrange a demo. Upon submitting a demo request on their official site, their team promptly responds within a single business day.
It’s even able to suggest custom workflows or automations that simplify the application process. Candidate experience is becoming critical in today’s recruitment marketing. With near full employment in many areas of the US, candidates have more options than ever before. As such, Chat PG Talent Acquisition leaders need to make it easy, simple, and engaging, during the candidate journey. Recruitment Chatbots can not only engage candidates in a Conversational exchange but can also answer recruiting FAQs, a barrier that stops many candidates from applying.
- In addition, it prioritises the best candidates by collecting the responses from the candidates and lessens the manual work for recruiters to do pre-screening calls.
- There are many aspects to consider, though one of the most important ones includes the selection of native integrations and the platform’s learning curve.
- They can engage candidates in meaningful conversations to understand their preferences, career aspirations, and work culture expectations.
- This consistency helps maintain a positive and professional image of the company, reinforcing its brand in the job market.
- In addition, the recruitment bot collects basic information such as the name, email ID, resume, and answers to the pre-screening questions from the applicants.
These virtual assistants, powered by advanced AI algorithms, are streamlining candidate screening, personalizing the recruitment experience, and increasing overall efficiency. Another innovative use case for self-service in recruitment is to improve the candidate experience. One common challenge when hiring is that candidates often feel like just a number—once they submit an application, they don’t really hear back from hiring companies unless they’re moving forward in the interview process. They can go a step further and assist candidates in finding the right job opportunities. By analyzing the candidates’ skills, qualifications, and preferences, chatbots can suggest suitable positions and guide them through the application process.
This can create a poor employer brand, which can negatively impact your recruitment efforts. You might also consider whether or not the platform in question enables the use of natural language processing (NLP) which makes up the base of AI chatbots. Indeed, for a bot to be able to engage with applicants in a friendly manner and automate most of your top-funnel processes, using AI is not necessary. You need to realize that not only there are hundreds of candidates competing for your position, but also, at the same time, there are numerous talent-hungry companies competing for the same pool of skilled applicants.
Paradox.ai is a major player in the HR tech space, so you’ve likely encountered them in your searches, conversations, and overall research. Their chatbot, named Olivia, uses natural language processing to have natural conversations with candidates, answer questions, and schedule interviews with recruiters. The AI Chatbot answers standard questions and upgrades applicants’ knowledge. It provides information to those who want to know more about the company (product, vision, values, and culture). It improves the candidate experience by providing answers immediately and offering 24/7 support.
Chatbot Resume: Stand out from the Crowd in 2022
This helps recruitment teams streamline their workflows considerably, and save on both time and resources. Recruitment chatbots are tools designed to answer questions mapped to preset answers from candidates applying for roles at your company, on behalf of your recruiting team. In the Jobvite 2017 Recruiting Funnel report, only 8.52% of career site visitors completed an application. That means that approximately 91% of candidates visited a career site and left without providing any contact information to contact them in the future.
They enhance efficiency, improve candidate experience, and support strategic decision-making in talent acquisition. By leveraging these versatile tools, businesses can optimize their recruitment processes, ensuring they attract and retain the best talent in a competitive market. Beyond answering queries, recruitment chatbots are programmed to interact with candidates actively. They can ask targeted questions to understand a candidate’s career aspirations, skills, and experiences, offering a more personalized interaction. This engagement helps in building a stronger connection with potential applicants, making them feel valued and heard.
Chatbots efficiently sift through applications, utilizing pre-set criteria to identify suitable candidates quickly. It expedites the initial selection process, saving valuable time that can be redirected towards more nuanced recruitment tasks. The use of artificial intelligence in recruiting is one of the most significant trends in talent acquisition.
Imagine a scenario where a job applicant visits a company’s career page and encounters a chatbot offering assistance with the application process. The chatbot uses natural language processing to ask relevant questions about the applicant’s qualifications, experience, and job preferences. Based on the responses, the chatbot filters and screens candidates, identifying those who meet the desired criteria and forwarding their profiles to recruiters for further review. Examples include recruitment chatbots deployed by companies like Unilever and L’Oreal, which automate initial candidate screening and enhance the efficiency of talent acquisition processes. A recruitment chatbot is an AI-powered tool that automates various aspects of the hiring process.

These chatbots assist with tasks like screening candidates, scheduling interviews, answering frequently asked questions, and enhancing candidate engagement. They use machine learning and natural language processing to interact in a human-like manner, offering a more efficient, consistent, and bias-free recruitment process. Navigating the digital recruitment landscape requires a balance of technology and human insight, and recruitment chatbots stand at this crossroads, offering a unique blend of efficiency and personalization.

Additionally, the platform seamlessly integrates with your Applicant Tracking System (ATS), eliminating the need for manual data entry in separate systems. AI-powered chatbots are more effective at engaging with candidates and providing a personalized experience. This means they’re able to update themselves, interact intelligently with users, and offer an overall candidate experience that is second to none.
They can engage candidates in meaningful conversations to understand their preferences, career aspirations, and work culture expectations. Finally, self-service tools can also be used to schedule follow-up interviews with candidates. This is a great way to keep candidates engaged throughout the recruitment process in real time and ensure that you don’t forget to follow up with them. No follow-ups, no acknowledgments of receipt, no way of asking questions about the job posting.
- As a recruiting team ourselves, we’re very much testing and exploring conversational AI (especially as we work at Dialpad!), and in this post, we’ll look closer at how traditional chatbots and conversational AI compare.
- Typical in-store recruiting messaging sends candidates to the corporate career site to apply, where we know 90% of visitors leave without applying.
- If you want a chatbot that can provide a more personal experience, an AI-powered chatbot may be a better choice.
- This continuous interaction fosters a positive impression of the company and keeps potential candidates interested.
To harness their full potential, integrate them thoughtfully into your hiring strategy. Begin by defining the chatbot’s role in your recruitment process, be it for initial candidate screening, scheduling interviews, or answering FAQs. Ensure it aligns seamlessly with your existing HR systems for a smooth workflow. Customize its interactions to reflect your company’s tone and values, making each candidate’s experience both personal and reflective of your brand. Regularly analyze the data and feedback it collects to refine your recruitment strategies.
This is a big reason why no-code conversational AI is quickly overtaking chatbots—it can learn on its own without that manual input. Once you’ve set up your chatbot, you can promote it to potential candidates through your company website and other digital channels like social media and SMS text messaging. Regardless of the job market, employers are always looking for new ways to improve the attraction and selection of talent. Bots are not here to replace humans but rather be the assistants you always wanted. In fact, if you don’t pick up the trend your candidates can beat you to it as CVs in the form of chatbots are gaining on popularity.
This initial screening helps create a shortlist of the most suitable candidates, thereby streamlining the selection process for human recruiters. Unlike traditional recruitment methods that require recruiters to go through countless resumes, AI can free human recruiters, who often spend 40 percent of their time sorting resumes. These include but are not limited to initial candidate screening, interview scheduling, answering frequently asked questions from applicants, creating job descriptions, and more. You can foun additiona information about ai customer service and artificial intelligence and NLP. They can answer questions, schedule interviews, and send reminders to candidates.
It aids in screening resumes, predicting candidate success, analyzing language in job descriptions for bias, and improving candidate matching through algorithms. AI also powers chatbots for immediate candidate interaction and data-driven decision-making, ensuring a more efficient, fair, and informed recruitment process. A more recent study shows that when chatbots for recruiting are involved on career sites, 95% more applicants become leads, 40% more of them complete a job application, and 13% more of them click ‘Apply’.
Even with an investment in a self-service tool powered by conversational AI, nothing can replicate the intuition and personal touch of a human recruiter. Automate repetitive tasks and free your team to spend more time with qualified talent. After all, the recruitment process is the first touchpoint on the employee satisfaction journey. If you manage to frustrate them before you hire them, they aren’t likely to last long.
While chatbots, automation and AI are fundamentally changing candidate communications, we believe that striking the right balance between personalized technology and human interaction is key to success. PeopleScout uses AI and other emerging technologies that personalize the candidate experience while also enabling our talent professionals to spend more time on critical functions. Employers should look for a talent partner with a comprehensive technology solution, where chatbots are just one piece of the puzzle. Through a chatbot, candidates can provide that same information in a conversational way that feels less daunting. In conclusion, AI Chatbots have emerged as a transformative force in the hiring process, revolutionizing recruitment strategies for employers.
They evaluate candidates based solely on their qualifications and experience, promoting a more equitable and diverse hiring process. It’s also important to recognize that not all chatbot technology is created equal. Low-quality technology could mean that a chatbot would have a hard time answering common questions or respond inappropriately. That would harm the employer brand even more than relying on slower, more traditional communication.
The most functionality comes with the purchase of the Paradox ATS, with limited or restricted functionality with many other common ATSs (this is especially true for those of you in the staffing & recruiting industry). Olivia is touted as integrating with some common vendors who may also be in your HR tech stack. A neat touch on their website is the ability to actually test out Olivia for yourself and see what the experience would be like for a candidate.
A recruiting chatbot brings “human interaction” back to the hiring process. It allows for a variety of possibilities to help you organize and streamline the entire workflow. It can easily boost candidate engagement and offer a frustration-free experience for all from the first touchpoint with your company. All that, while assessing the quality of applicants in real-time, letting only the best talent reach the final stages. Recruitment chatbots leverage AI algorithms to analyze candidate data and tailor interactions based on individual preferences and behaviors. AI in recruitment automates and optimizes various aspects of the hiring process.
Integrated with Chatbot API, these widgets offer a dynamic channel for two-way communication, ensuring a consistent and engaging experience for candidates. 66% of job seekers are comfortable with AI apps and recruitment Chatbots to help with interview scheduling and preparation, as found in a survey by The Allegis survey. However, hiring a chatbot eliminates this drawback by providing https://chat.openai.com/ instant and accurate answers to standard or frequently asked questions (FAQs). It responds to questions such as job description, location, or required critical skills in the job. If you’ve made it this far, you’re serious about adding an HR Chatbot to your recruiting tech stack. When you have a tight hiring funnel, talented candidates can quickly get lost in the sea of resumes.
During the course of my career, I have been both in the position of a job seeker and recruiter. Streamline hiring and achieve your recruiting goals with our collection of time-saving tools and customizable templates. Are you one of those hiring professionals who spend hours of time manually reviewing candidate resumes and segmenting applications… The tool supports the entire life cycle of the bots, from inventing and testing to deploying, publishing, tracking, hosting and monitoring and includes NLP, ML and voice recognition features.
The organisation was trying to remove the corporate perspective from the candidate experience and make it more candidate-centric. The conversion rate in the hiring was low due to the overly strict hiring process. Espressive’s solution is specifically designed to help employees get answers to their most common questions (PTO, benefits, etc), without burdening the HR team.
Through this engagement, they gain insights into your team’s specific challenges, subsequently arranging a customized demo session. Hence, there is no need to wait around wondering whether they have been communicating accurately based upon initial interactions via text message/WhatsApp once applied. It provides a modern, convenient way for candidates to communicate with recruiters and vice versa. ICIMS Text Engagement also offers a variety of features and capabilities, making it a valuable resource for organizations of all sizes. If you have any questions or concerns, be sure to get in touch with the chatbot’s customer support team.
Recruiting Automation is the process of studying the recruiting process steps required to hire an employee. Once the process is documented, the steps can be reviewed to determine which steps might be reorganized, removed, or automated, based on current needs and available technology and resources. Conduct assessments and interviews directly, whether it’s through direct assessments or asynchronous interviews. Our system takes care of rescheduling, reminders, and follow-ups, ensuring a smooth experience.
For example, a chatbot can take a customer’s order and process it without the need for a human agent. If you’re like most people, you probably think of chatbots as something that’s only used for customer service. However, chatbots can actually be used for a variety of different purposes – including recruiting. In a similar fashion, you can add design a reusable application process FAQ sequence and give candidates a chance to answer their doubts before submitting the application. In this section, we will present a step-by-step guide to building a basic recruitment chatbot. With the every evolving advancement of chatbot technology, the cost of developing and maintaining a bot is becoming more and more attainable for all types of businesses, SMBs included.
The post Recruitment Chatbots: A TA Leader’s Guide appeared first on Toppr-guides.