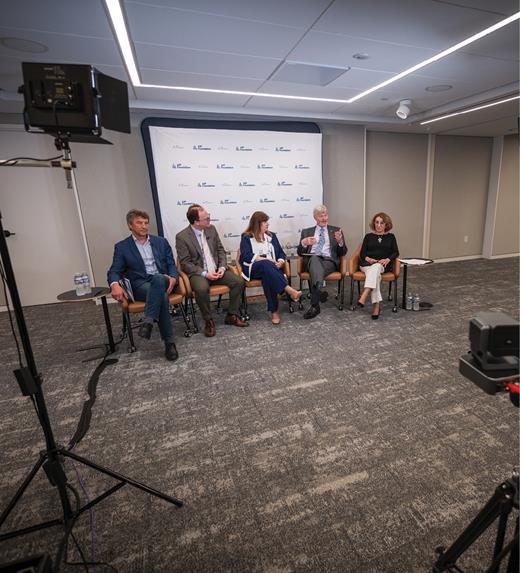
From left: Evgeni Gousev, Jesse Thaler, Valerie Browning, Walter Copan, and France Córdova, moderator.
ERIC STOCKLIN PHOTOGRAPHY
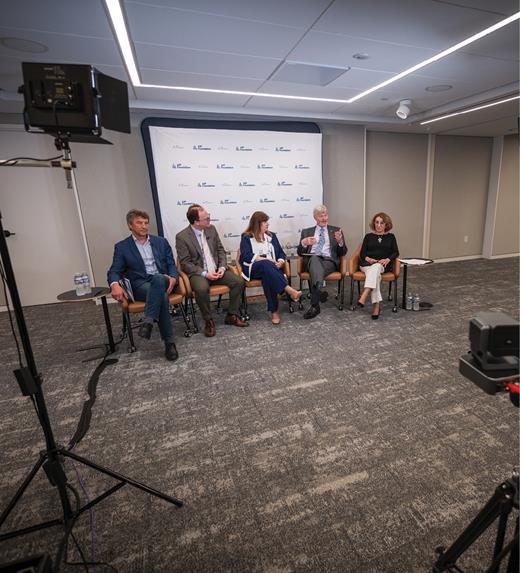
From left: Evgeni Gousev, Jesse Thaler, Valerie Browning, Walter Copan, and France Córdova, moderator.
ERIC STOCKLIN PHOTOGRAPHY
Close modal
Artificial intelligence and physics are changing one another, with implications that stretch from the classroom to the stars. The impacts can be seen across academia and industry, defense and security, granting and governance.
Leading physicists in academia, government, and industry came together earlier this year for a panel discussion to share their hopes for how machine learning and generative AI could transform discovery, creativity, intellectual property, training, communication, and the workforce. Convened by the AIP Foundation on 11 April 2024 at the American Center for Physics in Washington, DC, the event was chaired by former NSF director France Córdova. (The AIP Foundation is part of the American Institute of Physics, which publishes Physics Today.) She noted how AI is revolutionizing so many aspects of science: “Artificial intelligence is in everything, everywhere, all at once, like an artful phantom, or a worrisome one, depending on your point of view.”
The following text is adapted and condensed from the transcript of the event.
Córdova: How is AI changing physics research? Is it accelerating discovery or catalyzing incremental advances?
Jesse Thaler (MIT and NSF): I was skeptical in 2016 when people were talking about the deep-learning revolution. I’m a theoretical physicist, so I do “deep thinking” with my chalk and chalkboard. I’ve since realized how the time-tested strategies of physics can merge with machine-learning strategies for processing large data sets. That has led to many advances.
Here are just three examples from my institute. We’d like to understand the strong nuclear force. To that end, 10% of all open supercomputing resources in the US are now devoted to solving the equations of lattice quantum chromodynamics, which describe the strong interaction that binds quarks and gluons into protons and neutrons and then into nuclei. Some of my colleagues are using a kind of generative AI to do first-principles calculations of hydrogen and helium and march through the periodic table.
Neutrino physics is another strategic area that the US is investing in. An experiment is being built to send beams of neutrinos from Fermilab to a mine in South Dakota (see the article by Anne Heavey, Physics Today, July 2022, page 46). The detector relies on liquid-argon time-projection chambers that give exquisite access to information about neutrinos. We can’t process this complex data—and reliably reconstruct neutrino events—without AI.
Those are both examples of how AI can influence physics research. But how can physicists influence AI research? I like the example of grokking. This is when a machine-learning algorithm is running and running and not learning, then suddenly has an epiphany. To a physicist, that sort of abrupt change is a phase transition. And indeed, what happens in grokking is that a gas of information suddenly crystallizes into knowledge. A lattice structure emerges in the latent space of learning architecture. Physicists are uniquely positioned to understand this process.
I’m holding on for dear life as my junior colleagues drag me deeper and deeper into this world that I so distrusted at first. In 2016, two graduate students showed me their machine-learning paper. It was in direct competition with my work. I had toiled to do quantum field theory calculations, and here they were with some neural network. I told them all the things that were wrong with their paper: “It’s not interpretable. You don’t have the uncertainties. You don’t understand the concepts involved.” I thought they’d go work for someone else for their PhD. Instead, their theses basically addressed all my concerns.
This experience showed me how we should not just take AI off the shelf and use it as is. To adapt these methods for physics, we need to include all the things that we usually do in pursuing our high standards of scientific discovery.
Walter Copan (Colorado School of Mines): There are so many ways that I see artificial intelligence coexisting with physics research.
Take the hardware of big physics experiments. AI control systems are enabling setups that used to take graduate students months, such as the control of exquisite laser experiments on really complex optical tables, or how we shape beams and particle accelerators to deliver exactly what is needed at the energy distribution required.
Exoplanets are another great example. AI was at the heart of finding or validating many of the 5000 planets discovered so far. They’re normally spotted because of variations in the intensity of the signals coming from a star when a planet orbits it. Artificial intelligence is absolutely suited to that kind of pattern-recognition phenomenon in time.
Indeed, AI pattern recognition can speed physics research and the discovery process anywhere massive amounts of data are involved, such as at the Large Hadron Collider or what we anticipate from the Electron–Ion Collider experiments at Brookhaven National Laboratory.
Valerie Browning (Lockheed Martin): Now with large language models and generative AI, artificial intelligence is evolving from being a very valuable computational tool into more of a collaborator. That will further accelerate discovery. There is real promise in several areas: the intersection of quantum computing and machine learning, the discovery of new materials for renewable energy systems, and at the nexus of physics-informed neural networks and machine learning.
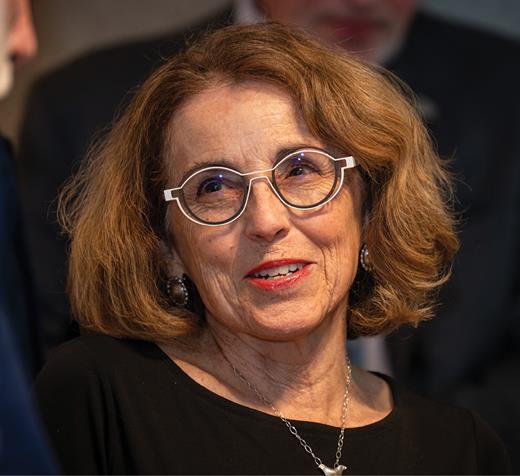
France Córdova
ERIC STOCKLIN PHOTOGRAPHY
Córdova: What is the perspective from industry?
Evgeni Gousev (Qualcomm Research and tinyML Foundation): In Silicon Valley, we develop leading-edge AI technologies, both hardware and software: all the tools used for scientific research. But developmentally, AI is at the toddler stage. You can teach a teenager to drive a car in about 10 hours. We’ve spent billions of dollars and more than a decade on autonomous driving, and it is not there yet. AI technology is still at the very beginning. Under the hood, it is running some basic probabilistic equations, doing matrix multiplication. This brute-force approach is not sustainable.
That’s where physicists can add value. We’re trained to solve problems and to connect dots. There are huge opportunities for us to help make AI more explainable, reasonable, reliable, and scalable.
Córdova: Are there risks for research?
Browning: Nothing about AI negates the need for due diligence and the scientific method. The risk comes in when you try to put things into practice. Models or insights that were developed using AI and machine-learning tools may have been valid in certain regimes or with certain constraints. There is risk when those caveats don’t flow into the engineering process.
In aerospace and defense, a mistake can be life-threatening. So a lot of what we do is to bring in that engineering rigor. Evaluation, validation, and verification is a challenge when you’re trying to anticipate all the edge cases—the rare events—that might arise in a very dynamic and potentially resource-constrained environment.
Copan: Risk is a trust-but-verify situation. Take AI and exoplanets again. The accuracy of the discovery process was over 96%. We can learn from what is not being predicted and from where we see false positives.
NIST has a key role with regard to standards for trustworthy AI. It is important for a range of applications to have test beds, where protocols for artificial intelligence can be validated and their accuracy can be verified independently.
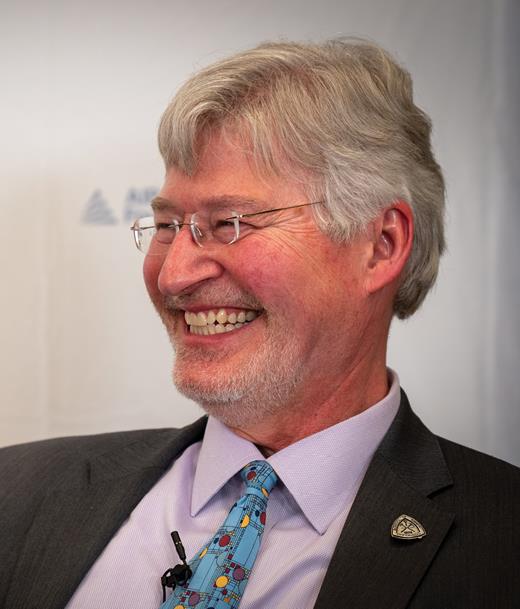
Walter Copan
ERIC STOCKLIN PHOTOGRAPHY
Córdova: How is AI changing education?
Thaler: On our campus, there’s a divide between people who are enthusiastic about AI and the ostriches who are putting their heads in the sand and saying that AI is not going to be relevant. But if we aren’t bringing these tools into the classroom, then we’re basically not doing our job as faculty to teach.
And once you force students to use generative AI, it really changes the type of exam you need to write. These days, a student can use a ChatGPT-style bot to answer a question about how to run, say, the kind of code that crunches data from the Laser Interferometer Gravitational-Wave Observatory. The chatbot can even generate example code. My MIT colleagues are developing a chatbot for scientific workflows. It is an incredible learning resource. Students who might not even know how to pose questions can interact with a chatbot to find out answers to things buried in the technical literature.
Copan: Artificial intelligence and machine learning are now the basic tools with which science is conducted. It is important that students become AI literate. They are the ones who are going to be advancing physics through AI and vice versa.
Córdova: Is AI altering science communication?
Thaler: Here, possibilities have grown in a surprising way, as I found out through an April Fools’ joke at my expense called ChatJesseT. It is a chatbot that knows all my papers, my Wikipedia entry, and my webpage. ChatJesseT speaks very enthusiastically about physics and AI. The students and postdocs at MIT built it using retrieval-augmented generation—drawing from a trusted corpus of text—plus some prompt engineering. This inspired us to train a chatbot on all of J. Robert Oppenheimer’s papers. It can give answers about technical concepts, such as the Born–Oppenheimer approximation, and about issues at the intersection of society and physics.
We found that the bot, Open-AI-mer, can spark a dialog with the public about the promise and perils of AI. Visitors to the Cambridge Science Festival were very interested to talk to Open-AI-mer and then to ask the physicist at the stand whether the bot’s responses were hallucinated or real.
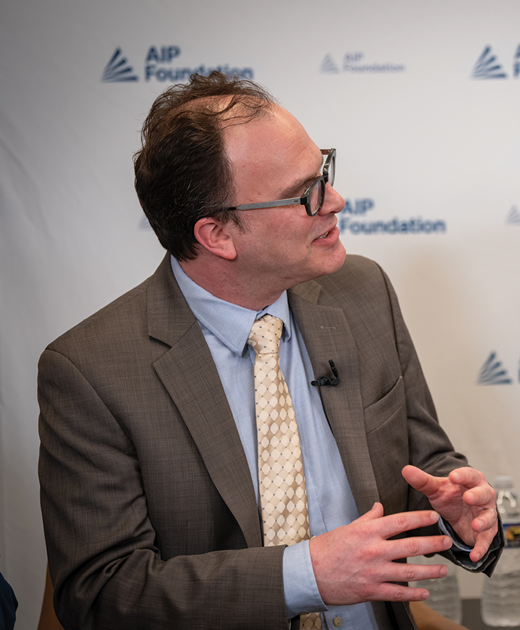
Jesse Thaler
ERIC STOCKLIN PHOTOGRAPHY
With my curmudgeon’s hat on, I used to think we’d never be able to use a language model for scientific discovery because the argot of physics is equations. I’ve come to appreciate that a ton of data is in the form of text. For instance, a high school student and a postdoc used AI with a database of Hubble images—it had the images from the Hubble Space Telescope and the proposals used to justify the telescope time. They put together the textual data and image data and created a new way of interacting with a scientific data set.
We physicists are realizing, perhaps to our chagrin, that language is actually a powerful means of communication. Generative AI can bring together technical experts and the public and create more opportunities for exchange.
Better still, we’ll be able to customize our own chatbots—ChatFrance, ChatWalt, if you will—to meet our needs. My wife is a lawyer. Her prompt-engineered version responds to certain types of legal questions in a format that’s useful for her professionally. Even if we don’t have programming expertise, we can program such tools to do various tasks that otherwise would be quite onerous.
Córdova: What are the key policy considerations for government funding agencies?
Browning: Approximately 70% of the roughly $4 billion budget that DARPA [Defense Advanced Research Projects Agency] is investing in R&D is either leveraging AI or advancing it.
Copan: We have seen a revolutionary shift in federal science and technology investment in AI. It naturally takes time for policy and regulation to catch up with the scale and pace of scientific discovery and technology. Now that the US wants to be the global leader in artificial intelligence, agencies will start to question if researchers are not using AI to achieve their results in a way that’s cost-effective and that ultimately develops scalable models that can be used for other purposes. Clearly, the opportunities across all science agencies will be tempered with the need to utilize the scientific process to validate models.
But the extent to which the US can capitalize on artificial intelligence as a force multiplier, as an enabler, and as a driver of efficiencies within the economy is also a workforce issue. There are gaps across the labor force that need filling, within and beyond the scientific enterprise, now and in the future. These have policy implications.
Córdova: Industry has most of the AI resources right now. Universities need these tools to pursue basic research questions. These tools are expensive and scarce. What’s the solution?
Browning: The challenge exacerbates the broader and longer-standing problem of inequities in high-power computing, including access to graphics processing units at our academic institutions. This hampers experiential learning. A student with access can work on some challenging real-world problems, and that experience can open doors that are shut to those who have fewer resources. Fixing this needs focus, consideration, and investment.
As a country, we recognize that access is a problem. The CHIPS and Science Act, for example, proposes big increases in funding to support greater access to STEM fields, including quantum and AI, and to strengthen research infrastructure and advanced computing. But there is more that needs to be done for today’s students.
Gousev: Part of the problem is that right now everything’s overhyped, from prices to order volumes. But AI is not a one-size-fits-all tool. Universities have to use it in a smart way, starting from the problem you’re trying to solve. The data in the cloud is pretty much already consumed by the GPT-type models. But there’s a lot more data in the real world. That’s what we need AI to collect and make actionable.
The hype is going to lessen. More capacity is coming as more startups enter the space to develop new approaches, innovations, techniques, and hardware. Algorithms will get more efficient. A human brain consumes 20 watts of power, and we can do very complex tasks. Graphics processing units can consume kilowatts—they’re super inefficient. I’m optimistic that AI is going to become more and more efficient and affordable.
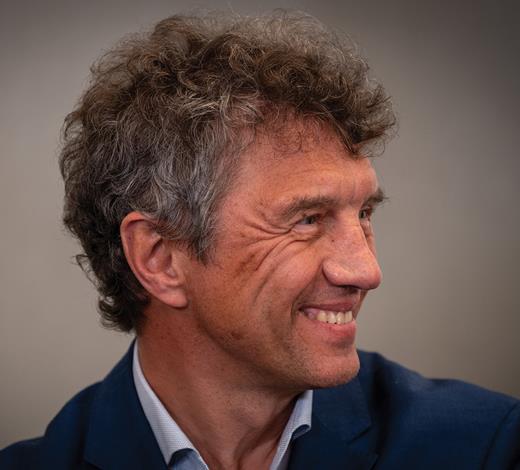
Evgeni Gousev
ERIC STOCKLIN PHOTOGRAPHY
Audience member: Are people with the right skills holding the reins of AI development?
Gousev: Now is a great time for physicists to shine. We’ve been a bit in our shells since a lot of physics discoveries were made in the 20th century. It’s time to come back. We can bring more explainability, efficiency, and common sense into the current chaotic world of AI.
Copan: In training physicists, we’ve got to work on the whole package and give them the ability to communicate persuasively and clearly so they can build consensus and teams. What is needed now is a combination of physics, business acumen, emotional intelligence, and communication skills: physics-plus.
Thaler: At our NSF AI institute, we are training incredibly talented interdisciplinary experts who go on to jobs in industry. They are top-notch problem solvers. Once you get those people on the ground floor of influential companies, they’re going to rise all the way to the top.
Audience member: What role does AI play in innovation and creativity?
Thaler: We don’t take enough advantage of the ability of computers to explore vast landscapes of possibilities. Part of me wonders whether some of the pinnacles of human achievement could have been reached through exhaustive search. Could Einstein’s relativity have come from an optimization principle? Did it really need the understanding of the geometry of spacetime? Was there some other way to get to that insight? Part of me thinks that we might have gotten lucky in the physical discoveries of the past. Things were simple and perturbative. Physicists could use basic rules to progress.
Maybe the problems we now face are intrinsically complex. Maybe physicists need to be careful about reducing things too far, to their simplest forms. Maybe we must embrace some level of complexity. Maybe for breakthrough physics insights, we need to start to think a little more like a computer and churn through many, many different options. Perhaps brute force is the future of creativity.
Copan: Experimental design and discovery are intrinsically human activities. We are in a very interesting symbiosis now with machines. There’s the patentable part of a discovery and the wild landscape of copyrightable work. What constitutes the beginning of one creative process, and what was the role of AI or of a machine-assisted program?
The US Patent and Trademark Office and other intellectual property offices around the world have made certain policy decisions about inventive activity and the role of the human inventor vis-à-vis machines. But in some ways, these are artificial constructs. It’s an evolving landscape.
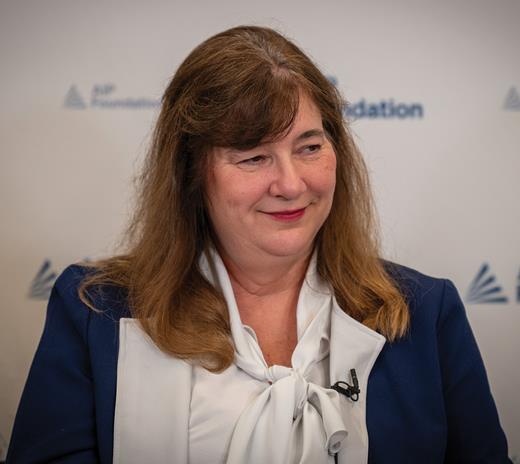
Valerie Browning
ERIC STOCKLIN PHOTOGRAPHY
Browning: There are examples in which AI has rediscovered laws of physics that we know. Who’s to say we’re not on the verge of AI discovering something new? And AI can explore lots of different options with just some prompts. Say I want to design a heat exchanger with particular properties but without the biases of what a heat exchanger looks like today. AI comes up with some pretty creative options. Pair that with new manufacturing techniques and materials, and I think there’s promise.
Córdova: We happen to have the father of the internet, Vint Cerf, in the audience. [Cerf is vice president and chief internet evangelist at Google and a recent trustee of the AIP Foundation.] It seems fitting to give him the last word.
Vint Cerf: It’s important to distinguish between general machine-learning models and specific large language models. The former have shown an ability to discover correlations that we might not have noticed. Noticing correlations is an important part of discovery in physics. With the large language models, I think we don’t fully appreciate what the hell is going on. We know that they are generative, and we know that they can hallucinate, but interestingly, they do bring together some unexpected juxtapositions as a result of the training methodology.
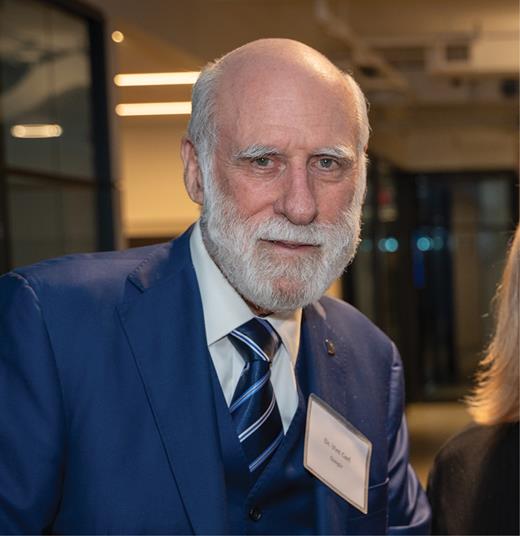
Vint Cerf
ERIC STOCKLIN PHOTOGRAPHY
The thing is that they don’t have enough context. I tested this by asking a large language model to write an obituary for me, and it generated a 700-word bio. It gave a date, which I thought was way too soon. It talked about my career. It gave me credit for stuff I didn’t do. It gave other people credit for stuff I did. It made up family members I don’t have.
This illustrates how large language models produce the verisimilitude of human discourse. They respond as if we had asked, “If you were a human being, what would you say to this prompt?” That’s all. But hiding within is some notion of knowledge because the statistics reflect real texts that have meaning. And so it can feel as if there’s a ghost in there that understands something.
Here’s a poignant example. One of our employees asked a chatbot to reverse a string of random characters. It produced the reverse string and added: “By the way, here’s a Python program that does that.” It stopped us in our tracks. Machine learning and generative AI are just tools for the most part. We have to be smart enough to distinguish between hallucination and vision.
Watch the full conversation at https://www.youtube.com/live/cUeEP15KN8M
A.
Heavey
,Phys. Today
75
(
7
),
46
(
2022
).
France Córdova is chair of the AIP Foundation Board of Trustees, president of the Science Philanthropy Alliance, and a former director of NSF. Valerie Browning is vice president of research and technology for Lockheed Martin’s Corporate Technology Office, and she is on the AIP Board of Directors. Walter Copan is vice president for research and technology transfer at the Colorado School of Mines and a former director of NIST. Evgeni Gousev is senior director of engineering at Qualcomm Research and board chair of the tinyML Foundation, and he is on the AIP Foundation Board of Trustees. Jesse Thaler is a professor of physics at MIT and director of the NSF Institute for Artificial Intelligence and Fundamental Interactions.